-
Notifications
You must be signed in to change notification settings - Fork 3.9k
New issue
Have a question about this project? Sign up for a free GitHub account to open an issue and contact its maintainers and the community.
By clicking “Sign up for GitHub”, you agree to our terms of service and privacy statement. We’ll occasionally send you account related emails.
Already on GitHub? Sign in to your account
kv: set sane default for kv.transaction.write_pipelining_max_batch_size #32606
Merged
craig
merged 1 commit into
cockroachdb:master
from
nvanbenschoten:nvanbenschoten/txnPipelineBatch
Nov 26, 2018
Merged
kv: set sane default for kv.transaction.write_pipelining_max_batch_size #32606
craig
merged 1 commit into
cockroachdb:master
from
nvanbenschoten:nvanbenschoten/txnPipelineBatch
Nov 26, 2018
Conversation
This file contains bidirectional Unicode text that may be interpreted or compiled differently than what appears below. To review, open the file in an editor that reveals hidden Unicode characters.
Learn more about bidirectional Unicode characters
tbg
approved these changes
Nov 26, 2018
There was a problem hiding this comment.
Choose a reason for hiding this comment
The reason will be displayed to describe this comment to others. Learn more.
Reviewed 1 of 1 files at r1.
Reviewable status:complete! 1 of 0 LGTMs obtained
Informs cockroachdb#32522. There is a tradeoff here between the overhead of waiting for consensus for a batch if we don't pipeline and proving that all of the writes in the batch succeed if we do pipeline. We set this default to a value which experimentally strikes a balance between the two costs. To determine the best value for this setting, I ran a three-node single-AZ AWS cluster with 4 vCPU nodes (`m5d.xlarge`). I modified KV to perform writes in an explicit txn and to run multiple statements. I then ran `kv0` with 8 DML statements per txn (a reasonable estimate for the average number of statements that an **explicit** txn runs) and adjusted the batch size of these statements from 1 to 256. This resulted in the following graph: <see graph in PR> We can see that the cross-over point where txn pipelining stops being beneficial is with batch sizes somewhere between 128 and 256 rows. Given this information, I set the default for `kv.transaction.write_pipelining_max_batch_size` to 128. Of course, there are a lot of variables at play here: storage throughput, replication latency, node size, etc. I think the setup I used hits a reasonable middle ground with these. Release note: None
9d7db78
to
52242a7
Compare
bors r+ Planning on backporting to 2.1. |
craig bot
pushed a commit
that referenced
this pull request
Nov 26, 2018
32606: kv: set sane default for kv.transaction.write_pipelining_max_batch_size r=nvanbenschoten a=nvanbenschoten Informs #32522. There is a tradeoff here between the overhead of waiting for consensus for a batch if we don't pipeline and proving that all of the writes in the batch succeed if we do pipeline. We set this default to a value which experimentally strikes a balance between the two costs. To determine the best value for this setting, I ran a three-node single-AZ AWS cluster with 4 vCPU nodes (`m5d.xlarge`). I modified KV to perform writes in an explicit txn and to run multiple statements. I then ran `kv0` with 8 DML statements per txn (a reasonable estimate for the average number of statements that an **explicit** txn runs) and adjusted the batch size of these statements from 1 to 256. This resulted in the following graph: 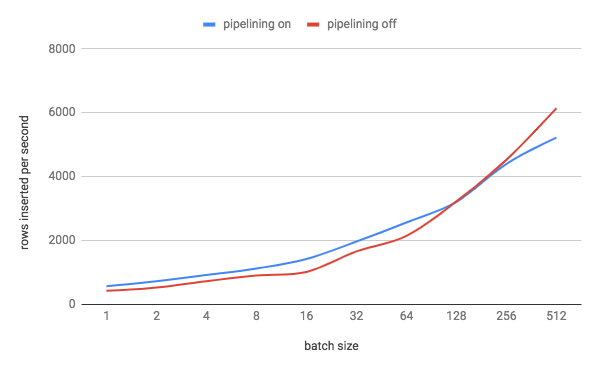 We can see that the cross-over point where txn pipelining stops being beneficial is with batch sizes somewhere between 128 and 256 rows. Given this information, I set the default for kv.transaction.write_pipelining_max_batch_size` to 128. Of course, there are a lot of variables at play here: storage throughput, replication latency, node size, etc. I think the setup I used hits a reasonable middle ground with these. Release note: None Co-authored-by: Nathan VanBenschoten <[email protected]>
Build succeeded |
Sign up for free
to join this conversation on GitHub.
Already have an account?
Sign in to comment
Add this suggestion to a batch that can be applied as a single commit.
This suggestion is invalid because no changes were made to the code.
Suggestions cannot be applied while the pull request is closed.
Suggestions cannot be applied while viewing a subset of changes.
Only one suggestion per line can be applied in a batch.
Add this suggestion to a batch that can be applied as a single commit.
Applying suggestions on deleted lines is not supported.
You must change the existing code in this line in order to create a valid suggestion.
Outdated suggestions cannot be applied.
This suggestion has been applied or marked resolved.
Suggestions cannot be applied from pending reviews.
Suggestions cannot be applied on multi-line comments.
Suggestions cannot be applied while the pull request is queued to merge.
Suggestion cannot be applied right now. Please check back later.
Informs #32522.
There is a tradeoff here between the overhead of waiting for consensus for a batch if we don't pipeline and proving that all of the writes in the batch succeed if we do pipeline. We set this default to a value which experimentally strikes a balance between the two costs.
To determine the best value for this setting, I ran a three-node single-AZ AWS cluster with 4 vCPU nodes (
m5d.xlarge
). I modified KV to perform writes in an explicit txn and to run multiple statements. I then rankv0
with 8 DML statements per txn (a reasonable estimate for the average number of statements that an explicit txn runs) and adjusted the batch size of these statements from 1 to 256. This resulted in the following graph:We can see that the cross-over point where txn pipelining stops being beneficial is with batch sizes somewhere between 128 and 256 rows. Given this information, I set the default for
kv.transaction.write_pipelining_max_batch_size` to 128.
Of course, there are a lot of variables at play here: storage throughput, replication latency, node size, etc. I think the setup I used hits a reasonable middle ground with these.
Release note: None